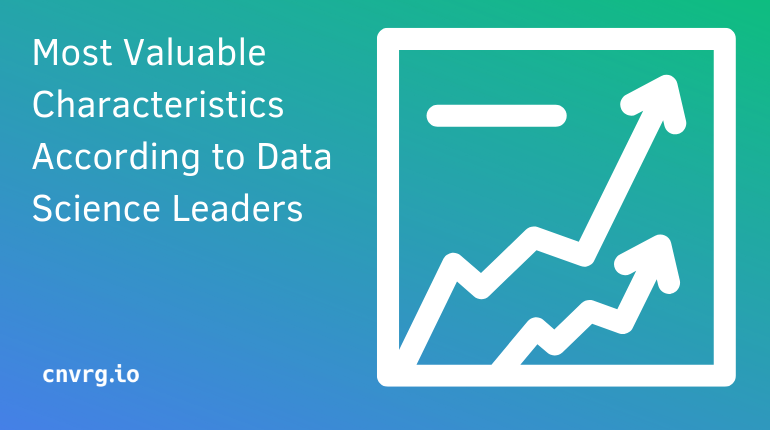
As a data scientist, there are endless things to learn or get better at. Developing skills in programming, computational fluency, advanced math ability, scientific methodology and even soft skills like communication and collaboration. All fields are important and ever evolving. The question is, in a team of data scientists, how do you create the most value for your company? What are the characteristics that make a data science valuable to a team?
Who better to answer this question than the data science leaders themselves. We rounded up answers from customers as well as other data science leaders from various industries to see what skills and characteristics they valued in data scientists on their team.
We asked the following:
Based on your experience leading data science teams, what is one skill or characteristic that makes a data scientist add the most value to a company?
Here's what the data science leaders had to say:
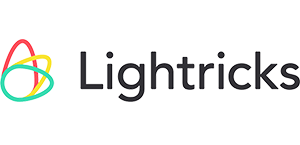
“The killer instinct of a data scientist is the most important thing. It is that part in them that allows them to follow through with tough tasks and complete the last stretch when 90% is already done. It’s the last 10% is all that really matters.”
Ofir Bibi, Director of Research at Lightricks
“The desire and ability to communicate,
“The one skill that adds the most value to a company, besides the obvious need to have a deep understanding of machine learning, is to have the ability to work in a production environment. This is a skill that is often lacking, but most needed.”
Bob
“One characteristic is being "hungry for data". While in other fields more workloads
Arthur Christian Cabatingan, Researcher/ Data Scientist at United Health Group | R&D.
"Communication is a key component to adding maximum value as a data scientist. Our most impactful data engineers clearly articulate results and commercial use cases to internal audiences with various degrees of technical ability."
Zach Flum, VP Franchise Analytics, Strategy & Technology at Goldman Sachs
“I can say that in our company (and in many others) it is very important to have a good mix of theoretical (scientific) skills together with hands-on software engineering skills and an understanding of business goals. In addition, it's important to be a good critic and to deeply understand the domain in which the algorithms operate. For example, you cannot be a good NLP Data Scientist without a deep knowledge and interest in texts and linguistics, and you cannot develop a good automatic recruitment algorithm without a good understanding of the HR/recruitment world and its challenges.” Oren Hazai, Chief Data Scientist at Woo.io
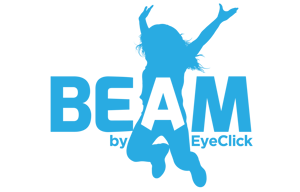
"I've learned over the years that without the knowledge of data scientists, there is no efficient way to turn cutting edge technology into actionable and digestible insights. With the knowledge and core Data Science skills like analysis and data processing, Big Data turns gigabytes of data into actionable insights that drive sales."
Nadav Shmuely VP of R&D at BEAM by EyeClick
What ultimately helps you add value as a data scientist is to fill the gaps that others can’t do. If you perform really well in your domain as a data scientist, then it makes you valuable to your company. As with any successful team, the bottom line is the bottom line. Your objective is to add business value, and by producing top performing models you are achieving that goal.
It’s becoming easier and easier to become a full-stack data scientist. As specialists arise such as data and machine learning engineers, people can master a specific technical domain. There are ample tools to help you fill in technical gaps and allow you to focus on the magic (algorithms).